Project Overview
Mathematica works with the National Center for Science and Engineering Statistics (NCSES) to automate its occupation coding process in the Survey of Doctorate Recipients (SDR) using advanced data-driven approaches and machine learning. This project aims to reduce the burden of manual review in the SDR and process the occupation coding timely and efficiently.
The NCSES currently relies on a labor-intensive review by trained coders to manually process more than 60 percent of occupation codes used in their survey. This process necessitated more than 2,800 coding-clerk hours for the 2015 and 2017 survey cycles combined. As the SDR captures new recipients of doctoral degrees in each survey cycle, the demand for manual review will continue to rise. NCSES sought a partner with machine learning capabilities that was familiar with their data who could explore new ways to automate occupation coding, reducing labor costs, and the potential for error.
SRI International
National Science Foundation
This occupation coding process is labor intensive. For example, in the 2015 and 2017 survey cycles, about 64 percent of the SDR responses required manual review, necessitating more than 2,800 coding-clerk hours according to an internal NCSES estimate. As the SDR captures new recipients of science, engineering, and health doctoral degrees in each survey cycle, the demand for manual review will continue to rise, increasing labor costs and the potential for human errors.
To reduce the burden of manual review and process the occupation coding timely and efficiently, NCSES asked Mathematica to explore ways to further automate occupation coding, using a data-driven approach that involves advanced analytic and machine learning (ML) methodologies. Mathematica developed an auto-coding algorithm that deploys a guidance-based (GB) module and an ML module, which is based upon results from the GB module, to automatically assign codes to SDR responses that currently require manual coding. This algorithm can cover a large proportion (percentage) of these SDR responses and performed well when tested with both 2015 and 2017 SDR data. Users can choose to deploy the GB module or the ML module separately. Specifically, using the ML module, the algorithm could automatically code more than 78 percent of the current manually coded records, with an accuracy of up to 96.3 percent compared with the current best codes determined by manual coders.
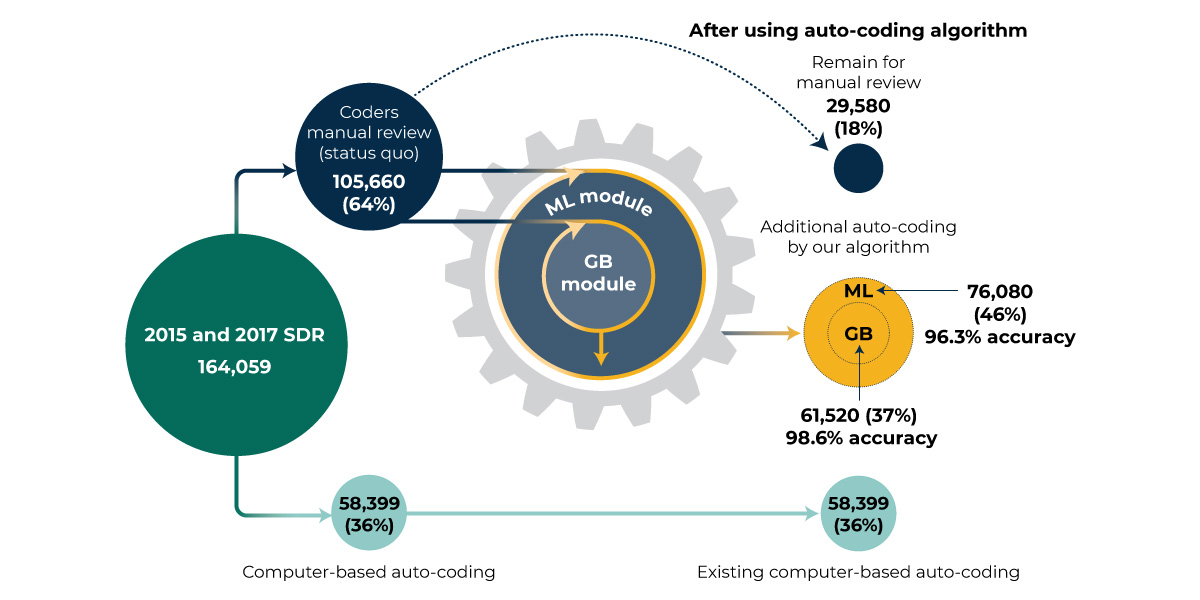
Machine-Learning Algorithm Saves More Than 2,000 Clerk Hours
Mathematica developed an auto-coding algorithm that has coded more than 78% of previously manually-coded SDR records, and:
- Saved NCSES more than 2,000 clerk hours.
- Coded with 96.3% accuracy when compared to manual coders’ best results.
Related Staff
See Clearly. Act Quickly.
From local to global challenges in health, human services, and international development, we’re here to improve public well-being and make progress together. Learn more about becoming a Mathematica client or partner.
Work With Us