Artificial intelligence (AI) holds great promise for improving health care and public health. By leveraging and processing large amounts of data at far greater speeds than humans, AI can generate predictions that can inform policy or treatment decisions. But as predictive algorithms in medicine and public health increase and the fields rely on them more, policymakers, data scientists, ethicists, and industry leaders must work together to develop best practices for addressing algorithmic bias. Algorithmic bias occurs when AI tools systemically make predictions that are discriminatory against groups of people. The potential problems stemming from algorithmic bias are well documented. For instance, as a result of algorithmic bias, a former hiring algorithm that Amazon used taught itself that male applicants were preferable to women. This was because the data used to develop the algorithms showed that most people who submitted resumes for software developer jobs were males. To inform discussions about how to address algorithmic bias, we are sharing lessons we learned from our participation in a national AI competition.
In the Centers for Medicare & Medicaid Services AI Health Outcomes Challenge, companies from the health care and AI communities demonstrated how AI tools could help prevent patient health events, such as unplanned hospital admissions and mortality, for Medicare beneficiaries. Specifically, by identifying patients at high risk for an unplanned hospital admission or death, and by providing information on the factors that contribute to a patient’s overall risk at the point of care, providers could work to address those factors. This, in turn, could decrease patients’ risk and ultimately improve their health. In addition, these predictions, if accurate, could ultimately inform new Centers for Medicare & Medicaid Services Innovation Center payment and service delivery models. As part of the challenge, Mathematica and six other finalists (out of an initial pool of more than 300 applicants) outlined how they addressed algorithmic biases that could impact risk predictions. Here’s what we learned, and how the Centers for Medicare & Medicaid Services and health decision makers can move forward to address algorithmic bias.
Identify where the biases exist in data so those biases can be addressed through modeling.
It is important to consider biases that might be reflected in the data sets used to develop health care AI tools. Prior work has demonstrated that the ramifications of these biases, if not addressed, can be dangerous. For example, recently Ziad Obermeyer and his colleagues found that a widely used AI tool was predicting that White patients who had the same clinical conditions as Black patients needed more health care services because, in the data set they used to develop the tool, White patients had historically received more services than Black patients and had higher health care costs. Obermeyer and his colleagues “estimated that racial bias [reduced] the number of Black patients identified for extra care by more than half.”
For the AI Challenge, we primarily used claims data and data related to social determinants of health (described below), to develop our AI tools. Previous research demonstrates that there are regional variations in the availability of health care services and providers who accept Medicare fee-for-service plans throughout the United States. In areas with greater availability, Medicare beneficiaries have easier access to health care, so many use more services and thus have more claims. In addition to regional variations in the use of health care services, previous research has shown evidence of racial bias in the rates at which chronic conditions are diagnosed that affect Medicare beneficiaries, including renal disease, cardiovascular disease, and cancer. Racial and ethnic minorities with these conditions are less likely to be diagnosed than White beneficiaries. To account for these biases that are reflected in claims data, we included beneficiaries’ state and county as model features as well as race and ethnicity.
Build diverse teams to create AI tools that are useful to end users.
When building algorithms, developers adjust what features the model will include and what the outputs they think will be the most important to display for end users. The process of selecting features and outputs can be another source of algorithmic bias because the features determine what information the model uses to make predictions and the outputs determine what information end users have to make decisions.
To ensure that we selected appropriate features and displayed the model outputs in a way that helps providers, we assembled a diverse team, partnered with other organizations, and engaged a wider community at Mathematica. Our team was diverse in terms of subject matter expertise, gender, age, race, and geographic location. Mathematica also partnered with physicians at The Health Collaborative, a data-driven nonprofit health care organization, and patient advocates at Patient Advocate Foundation in multiple rounds of iterative discussion about our prototype user interface to improve its usefulness. The team building the models and interfaces also engaged the wider clinician community at Mathematica through human-centered design exercises to gather feedback on the prototypes and had discussions on the features to include in the algorithms. For instance, the team had clinicians use ranked choice voting to determine the clearest plain language representation of risk scores on the user interface. From these discussions and exercises, our solution implemented feedback and promoted trust by presenting plain language representations of risk scores, an easy-to-understand list of factors driving the risk calculations, and direct language for limitations to our models and data.
Another way to account for and address biases in building algorithms would be to record discussions and maintain a decision log on how and when model features and outputs are decided.
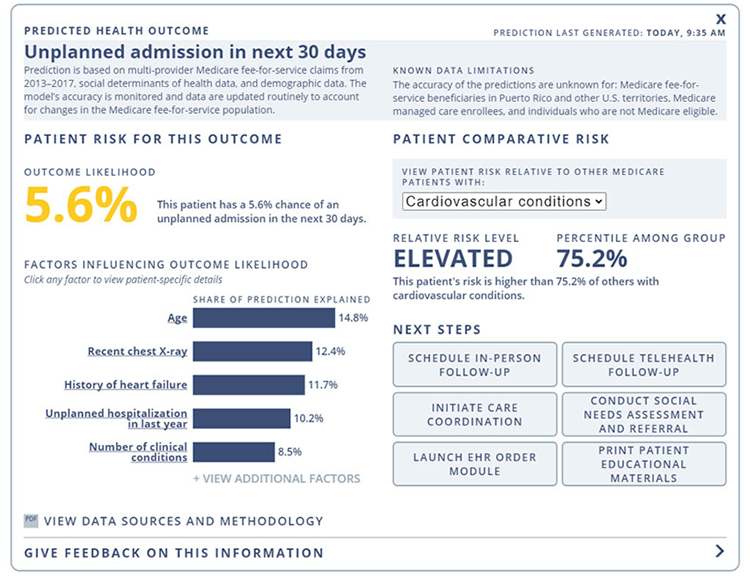
Above is an example of the visual display, informed by human-centered design principles and stakeholder feedback, that health care clinicians would see when using the AI tool developed by Mathematica and its partners.
Think critically about data cleaning and how to address missing data to minimize the risk of introducing bias in the first place.
Using data sets that suffer from quality issues or that have significant missing data can introduce bias into prediction tools. Our approach to cleaning our data included de-duplicating claims and beneficiaries to ensure that each beneficiary encounter only contributed once to each model. We also removed claims paid by managed care organizations because not all claim types paid by Medicare Advantage plans are captured in the limited data set used to train our models.
Issues associated with missing data are especially problematic when data are not missing at random, such as when data for one group of people are more likely to be missing than data for other groups of people. It is important to investigate the upstream workflows and data collection methodologies that could be yielding missing data.
In our case, the most important features for our AI models did not have missing data because they were derived from claims data. Several of our features, however, were derived from other data sets and did have missing data. In an extreme case, 23 percent of data was missing for the “number of days that air quality is measured” feature that we planned to include in our AI tools. Looking further upstream at the data source for this feature, the EPA Air Quality Index Report, it is possible that there was a high missingness rate for this feature because metropolitan statistical areas of fewer than 350,000 people were not required to report their air quality index. In other words, there could be higher rates of missing air quality index data from rural areas. Because of the high amount of missing data, we ultimately decided not to include that feature in the final tools out of concern that we might introduce substantial bias. We also found that about 1 percent of data was missing for another feature we included in our models: the Outpatient Dialysis Facility Per User Actual Costs feature. In this instance, we decided to retain that feature because the amount of missingness was low and the feature did not significantly contribute to the AI tools.
Account for differences in race and ethnicity between the training data and the population to which you will apply the AI tool.
We compared our training data with information on the entire Medicare fee-for-service population during the same time period to make sure it was similar in terms of age, gender, race, and ethnicity distribution. We found some differences in race and ethnicity: Our data had a slightly higher percentage of White beneficiaries and a slightly lower percentage of Hispanic beneficiaries. We accounted for differences in race and ethnicity by reweighting all observations (adjusting the level of influence or importance of a variable or observations) in our data to more closely reflect the overall race and ethnicity distribution of the Medicare fee-for-service population by year.
Check your work to confirm that AI tools are accurate for important patient subgroups.
After developing our AI tools, we examined their accuracy for different subgroups based on race, age, gender, and clinical conditions. We confirmed that the tools performed equally well across racial and ethnic subgroups as well as equally well across important clinical subgroups. Accuracy was slightly lower, however, among people older than age 79, possibly because of less observations in this subgroup. Although the unplanned admission model performed equally well across genders, the mortality model performed slightly better for females. Despite these minor differences, our findings made us confident that our AI tools were providing high quality predictions across subgroups.
Charting a path forward
We believe the steps we took helped ensure that our AI tools provided accurate predictions for Medicare fee-for-service beneficiaries regardless of their demographic characteristics. Yet work to address algorithmic bias does not end after an AI prediction tool is developed. Predictive algorithms implemented in a clinical setting must be monitored over time to examine whether those tools still provide accurate predictions as clinical care and demographic trends change. Specifically, approaches such as calibration drift detection systems help monitor the performance of AI tools over time and help teams adjust the algorithm to account for shifts in performance. We hope others can learn from our experience, and we look forward to opportunities to learn as well. Research and co-creation with end users are critical to promoting best practices for addressing algorithmic bias when building, training, validating, and monitoring the health of health care AI tools.